Artificial Intelligence
That’s funny – but AI does not get the joke
Researchers challenged AI models and humans to match jokes to cartoons and explain the joke. Guess who won?
Large neural networks, a form of artificial intelligence, can generate thousands of jokes along the lines of “Why did the chicken cross the road?” But do they understand why they’re funny?
Using hundreds of entries from the New Yorker magazine’s Cartoon Caption Contest as a testbed, researchers challenged AI models and humans with three tasks: matching a joke to a cartoon; identifying a winning caption; and explaining why a winning caption is funny.
In all tasks, humans performed demonstrably better than machines, even as AI advances such as ChatGPT have closed the performance gap. So are machines beginning to “understand” humor? In short, they’re making some progress, but aren’t quite there yet.
“The way people challenge AI models for understanding is to build tests for them – multiple choice tests or other evaluations with an accuracy score,” said Jack Hessel, Ph.D. ’20, research scientist at the Allen Institute for AI (AI2). “And if a model eventually surpasses whatever humans get at this test, you think, ‘OK, does this mean it truly understands?’ It’s a defensible position to say that no machine can truly `understand’ because understanding is a human thing. But, whether the machine understands or not, it’s still impressive how well they do on these tasks.”
Hessel is lead author of “Do Androids Laugh at Electric Sheep? Humor ‘Understanding’ Benchmarks from The New Yorker Caption Contest,” which won a best-paper award at the 61st annual meeting of the Association for Computational Linguistics, held 9-14 July in Toronto.
Lillian Lee, the Charles Roy Davis Professor in the Cornell Ann S. Bowers College of Computing and Information Science, and Yejin Choi, Ph.D. professor in the Paul G. Allen School of Computer Science and Engineering at the University of Washington, and the senior director of common-sense intelligence research at AI2, are also co-authors on the paper.
For their study, the researchers compiled 14 years’ worth of New Yorker caption contests – more than 700 in all. Each contest included: a captionless cartoon; that week’s entries; the three finalists selected by New Yorker editors; and, for some contests, crowd quality estimates for each submission.
For each contest, the researchers tested two kinds of AI – “from pixels” (computer vision) and “from description” (analysis of human summaries of cartoons) – for the three tasks.
“There are datasets of photos from Flickr with captions like, ‘This is my dog,’” Hessel said. “The interesting thing about the New Yorker case is that the relationships between the images and the captions are indirect, playful, and reference lots of real-world entities and norms. And so the task of ‘understanding’ the relationship between these things requires a bit more sophistication.”
In the experiment, matching required AI models to select the finalist caption for the given cartoon from among “distractors” that were finalists but for other contests; quality ranking required models to differentiate a finalist caption from a nonfinalist; and explanation required models to generate free text saying how a high-quality caption relates to the cartoon.
Hessel penned the majority of human-generated explanations himself, after crowdsourcing the task proved unsatisfactory. He generated 60-word explanations for more than 650 cartoons.
“A number like 650 doesn’t seem very big in a machine-learning context, where you often have thousands or millions of data points,” Hessel said, “until you start writing them out.”
This study revealed a significant gap between AI- and human-level “understanding” of why a cartoon is funny. The best AI performance in a multiple choice test of matching cartoon to caption was only 62% accuracy, far behind humans’ 94% in the same setting. And when it came to comparing human- vs. AI-generated explanations, humans’ were preferred roughly 2-to-1.
While AI might not be able to “understand” humor yet, the authors wrote, it could be a collaborative tool humorists could use to brainstorm ideas.
Other contributors include Ana Marasovic, assistant professor at the University of Utah School of Computing; Jena D. Hwang, research scientist at AI2; Jeff Da, research assistant at the University of Washington Rowan Zellers, researcher at OpenAI; and humorist Robert Mankoff, president of Cartoon Collections and long-time cartoon editor at the New Yorker.
The authors wrote this paper in the spirit of the subject matter, with playful comments and footnotes throughout.
“This three or four years of research wasn’t always super fun,” Lee said, “but something we try to do in our work, or at least in our writing, is to encourage more of a spirit of fun.”
This work was funded in part by the Defense Advanced Research Projects Agency; AI2; and a Google Focused Research Award.
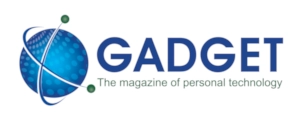