Artificial Intelligence
New AI tool dives deeper into sleep
Researchers at Mount Sinai have developed a transformer-based tool to analyse sleep stages more accurately, using over 1-million hours of sleep data.
A powerful new AI tool has been developed to analyse an entire night’s sleep. Built on the same transformer architecture used in large language models such as ChatGPT, the tool was created by researchers at the Icahn School of Medicine.
The findings are presented in a study that analysed 1,011,192 hours of sleep. Titled A foundational transformer leveraging full night, multichannel sleep study data accurately classifies sleep stages, the research was published in an online issue of the journal Sleep.
The model, called Patch Foundational Transformer for Sleep (PFTSleep), analyses brain waves, muscle activity, heart rate, and breathing patterns to classify sleep stages more effectively than traditional methods, streamlining sleep analysis, reducing variability, and supporting future clinical tools to detect sleep disorders and other health risks.
Current sleep analysis often relies on human experts manually scoring short segments of sleep data or using AI models that are not capable of analysing a patient’s entire night of sleep.
The new approach, developed using thousands of sleep recordings, takes a more comprehensive view. By training on full-length sleep data, the model can recognise sleep patterns throughout the night and across different populations and settings, offering a standardised and scalable method for sleep research and clinical use.
“This is a step forward in AI-assisted sleep analysis and interpretation,” says first author Benjamin Fox, a PhD candidate at the Icahn School of Medicine at Mount Sinai in the artificial intelligence and emerging technologies training area.
“By leveraging AI in this way, we can learn relevant clinical features directly from sleep study signal data and use them for sleep scoring and, in the future, other clinical applications such as detecting sleep apnea or assessing health risks linked to sleep quality.”

Image courtesy Benjamin Fox.
The model was built using a large dataset of sleep studies (polysomnograms) that measure key physiological signals, including brain activity, muscle tone, heart rate, and breathing patterns.
Unlike traditional AI models, which analyse only short, 30-second segments, this new model considers the entire night of sleep, capturing more detailed and nuanced patterns.
The model is trained via a method known as self-supervision, which helps learn relevant clinical features from physiological signals without using human labelled outcomes.
Co-senior author Ankit Parekh, PhD, assistant professor of medicine and director of the Sleep and Circadian Analysis Group at Mount Sinai, says: “Our findings suggest that AI could transform how we study and understand sleep. Our next goal is to refine the technology for clinical applications, such as identifying sleep-related health risks more efficiently.”
The researchers say that this AI tool, while promising, would not replace clinical expertise. Instead, it would serve as a powerful aid for sleep specialists, helping to speed up and standardise sleep analysis.
The team’s research aims to expand its capabilities beyond sleep-stage classification to detecting sleep disorders and predicting health outcomes.
Nadkarni, MD, MPH, co-senior author and chair of AI and Human Health at Mount Sinai, says: “This AI-driven approach has the potential to revolutionise sleep research. By analysing entire nights of sleep with greater consistency, we can uncover deeper insights into sleep health and its connection to overall well-being.”
He is the inaugural chief of the Division of Data-driven and Digital Medicine and co-director of the Mount Sinai Clinical Intelligence Center.
The study’s authors, as listed in the journal, are Benjamin Fox, Joy Jiang, Sajila Wickramaratne, Patricia Kovatch, Mayte Suarez-Farinas, Neomi A. Shah, Ankit Parekh, and Girish N. Nadkarni.
* Read the paper here.
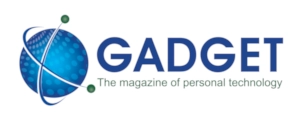