Artificial Intelligence
Dependable data will ‘keep Artificial Intelligence safe’
Latent potential will remain untapped unless organisations can safeguard their AI investments, writes VALENCIA KARAGEORGIADES, technology architect at SAP Africa.
Generative artificial intelligence (AI) is one of the most significant leaps forward for the power of technology and its impact on every sphere of our lives.
As organisations increasingly embrace generative AI to derive insights, automate processes, and drive innovation, the foundation of accurate and well-organised data within their business systems becomes pivotal for realising the full potential of AI applications.
The IDC forecasts that spending on GenAI will reach $143-billion by 2027, growing at a CAGR of more than 73%. Much of the spend will be directed to GenAI business cases where productivity boosts are paramount. One McKinsey study found that GenAI could add the equivalent of $2.6-trillion to $4.4-trillion in productivity gains annually.
However, much of this latent potential will remain untapped unless organisations can safeguard their AI investments with accurate, dependable data. There is an indispensable connection between the correct data setup in enterprise systems and the success of generative Artificial Intelligence (AI).
Companies seeking to leverage AI as a long-term value driver must therefore ensure they maintain trust and integrity in the data powering their algorithms.
Trust drives AI value
Trust is a critical component of responsible AI adoption. By utilising reliable data, organisations can build and maintain trust with users and stakeholders, fostering a positive relationship between AI technology and society.
Trust is also important for accurate real-time decision making. In today’s dynamic and fast-paced business landscape, organisations and leaders need to make informed decisions based on accurate data-driven insights. By aligning the outcomes of analytics processes with their own strategic objectives, business leaders are better able to take optimal action and steer the company through adversity, or take advantage of emerging opportunities.
It is therefore important that the data is indeed reliable to prevent erroneous outputs which could be costly and negatively impact an organisation and its reputation. When users trust the output of generative AI applications, the business benefits from higher levels of collaboration and innovation.
To build trust and mitigate risks associated with AI adoption, companies need dependable data that holds the necessary insights and avoids bias. This increases the likelihood that businesses and end-users take the calculated risks necessary for broader AI deployment.
Advantages of data-driven business strategies
To drive greater adoption of AI solutions, organisations must continuously improve their data strategies. This provides several advantages to the success of the business, including:
Greater competitiveness: Organisations with a good data strategy gain a competitive edge when their high-quality data is partnered with powerful AI algorithms to produce better strategic choices and business outcomes.
Increased operational efficiency: Well-managed and high-quality data promotes streamlined business processes. When data is accurate and readily available, operations can more easily be optimised, resulting in cost efficiencies .
Improved innovation and scalability: By leveraging insights built on a good data strategy, businesses can identify market gaps, explore new opportunities, and create products or services that meet evolving customer needs faster than their competitors.
Organisations must also not underestimate the impact of transformative data innovations that help them harness the full power of their data to drive deeper insights, faster growth and more efficiency in the era of AI. The introduction of new data innovations enable organisations to deliver meaningful data to every data consumer with business context and logic intact – enabling them to adapt faster to market changes and make more efficient decisions. The newly-launched SAP Datasphere solutions, for example, mobilise an extensive global partner ecosystem to enable organisations to transform their business data fabric architecture, allowing them to access insights from their data wherever it resides.
Key elements of an AI-ready data strategy
Organisations that consistently unlock business value from their data-driven strategies have a few characteristics in common. The key elements to enhancing data strategies for AI readiness include:
Strong alignment with business objectives: A well-defined data strategy aligns with the overall goals and objectives of the organisation by establishing a clear roadmap for leveraging data to meet business requirements and objectives.
Effective data governance: Establishing data governance frameworks within the data strategy ensures that data is treated as a valuable asset. Clear ownership, accountability, and data stewardship protocols lay the foundation for maintaining data integrity and consistency.
The highest levels of data quality: The accuracy and reliability of digital transformation and related AI implementations hinge on the quality of the data being input into the system. Incorrect or inconsistent data at the outset can lead to errors and inefficiencies, extending project timelines and adding layers of additional costs and complexity.
The use of industry best practices: Following industry best practices for data setup ensures compatibility and optimal utilisation of the modules being implemented at lower cost and with less complexity. Adhering to recommended data structures, field configurations, and master data management principles is essential for a seamless project in support of AI readiness.
A careful and considered data migration: Proper data setup involves meticulous planning for data migration from legacy systems to the new system. This includes data cleansing, transformation, and validation processes to ensure a smooth transition without compromising data integrity.
Achieving AI readiness demands a holistic approach to data setup, data strategy, and governance. Organisations that prioritise these guidelines will be better positioned to unlock the full potential of AI, foster innovation, and maintain the highest ethical standards.
By adhering to these principles, businesses can navigate the complexities of AI deployment responsibly and contribute positively to societal and economic advancements.
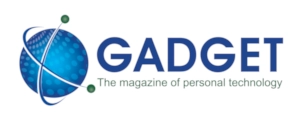